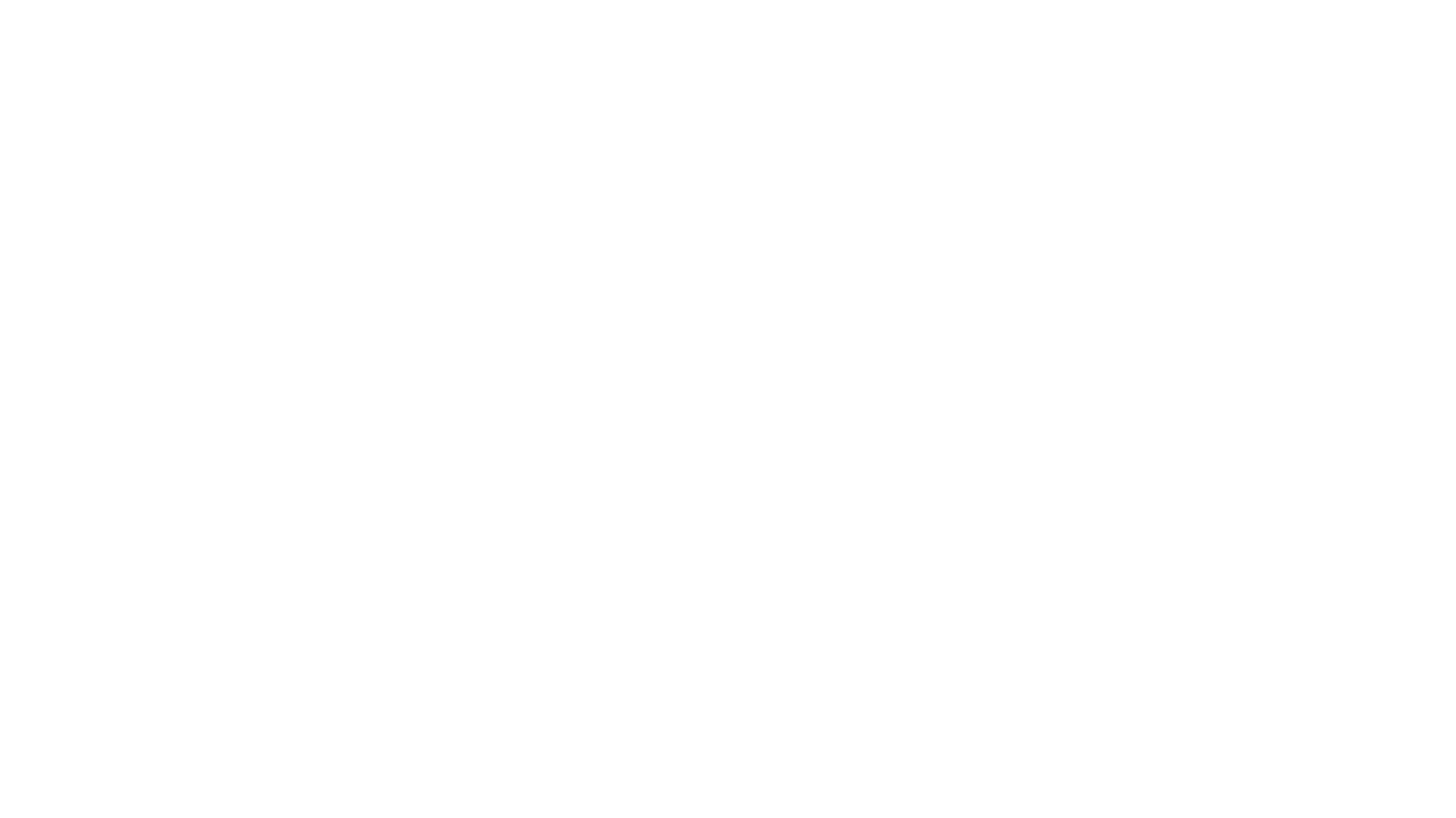
Big things are coming soon, but it is because of the annoying squeaky wheels…
Big action only happens from Rule Followers in response to Rule Breakers, so you are the primary driving force for change. It’s never fun being a martyr, or watching well meaning folks get crucified by rules upon a wall of No’s. It sucks, it’s demoralizing, and it burns people out. But that sacrifice does have great impact, though usually just not directly.
The secret knowledge hiding in plain sight,…and it’s as easy to find as a belt buckle.
You can reverse engineer the best leads for technology collaboration, partnerships, and sales from public defense contracting data. All the RFPs and approved contracts for defense organizations big and small are publicly available. Want to know what the AI priorities are, and who is funding them? Want to know the trends in AI applications for DoD? All this and more is not hard to figure out, and modern Generative AI tools make this easier than ever.
You keep asking me for it...so here's the answer & opportunity .
"What are the DoD Generative AI Needs?"I gets asked this question repeatedly at least once a day. Instead of me just giving you a hand wavy response, let's do something different.
Let me just go ahead and upset everyone, everywhere, all at once…
A RIDICULOUS war rages in online communities across social media over DoD GenAI software development. The gnashing of teeth across Internet enclaves of DoD members and defense contractors yesterday seemed to reach a new fever pitch. With more folks publicizing internally developed DoD projects like NIPRGPT, and the inevitable drumbeat of defense contractor backlash for non-commercial development was quite loud. It’s all a bit much at this point, so I think it’s important to level set some expectations for folks who either forgot or haven’t learned yet from experience.
Generative AI is starting to piss me off…
I’m getting really tired of seeing things I really want to buy, but are apparently just visualizations of someone’s very genius Generative AI musings.
Just a beautiful video on the history of Large Language Model development…
Hope everyone has a wonderful holiday season. Plenty of time to relax, decompress, and spend time with loved ones. Invariably I always find myself in the random conversation with friends and family about, “what is it exactly that you do?”
What could a LLM-based data refinery look like?
Not a one size fits all solution, but rather just the map of the possible for what an automated data refinery could be. Remember like the physical commodities refineries, the goal of each process is to go from raw to processed, not raw to perfect. Use cases are unique, and require the right tools.
GPT-4V image analysis use case…that’s VERY controversial…
Another example of using GPT-4V to perform image analysis. Bonus points for those who figure out what/where this image is depicting (and why that makes the results controversial).
Handwriting analysis test with GPT-4V…
Using GPT4 to first construct a complex prompt for handwriting analysis, then using GPT-4V to execute the prompt with the handwriting samples. Truly fascinating example of what you can do with this model, and the high degree of accuracy and detail you can produce with a complex use case.
Setting up the dominos…how Generative AI can enable the streamlining of reporting.
Every person in the Air Force has a series of interlinked personnel based activity reports that they are either required or optionally available to them relative to performance. These reports have an interlinked position in time and scope, each building from the previous, and supporting the next in the chain. A simple example would be personnel performance. At the end of the chain you could have a medal package for the award in the recognition of achievement, and you could follow that record of action that justified the achievement back through a series of documents each decreasing in scope and time, all the way down to the smallest and shortest form for weekly activity tracking.
The most abundant and yet untapped trove of valuable data is in your mind.
With so many people making the pilgrimage to the DC area for AFA this week, I thought this would be a timely topic to bring up. Remember that LLMs fundamentally rely on mass amounts of data to function well. As good as scraping the entirety of the internet is, I think we can all agree that’s neither authoritative nor necessarily as deep or accurate as any topic actually goes. So where are the most detailed and accurate sources of knowledge on any particular domain? In the minds of the people that work in those domains everyday.
Some random notes that didn’t really fit neatly into the study findings…
Unlike my four previous posts on the topic of our AI study on LLM technology, this one is more a collage of items that didn’t really fit well enough to include in the written presentation. They might come up in a verbal presentation, but not likely unless a question drove the interest in a deep dive. But I like having some sort of written record beyond just my endless pages of random notes, so I thought this might make an interesting post to share.
Lessons learned from LLM based Chatbot study and assessment…#4
Continuing on in the series of lessons learned from the chatbot study, let’s look now into the art of prompt engineering.
Lessons learned from LLM based Chatbot study and assessment…#3
Continuing on in the series of lessons learned from the chatbot study, next let’s discuss use case discovery.
Lessons learned from LLM based Chatbot study and assessment…#2
Continuing on in the series of lessons learned from the chatbot study, we find a clear but important distinction.
Lessons learned from LLM based Chatbot study and assessment…#1
It is time to conclude the AI study focusing on LLM-based Chatbots I have been leading as my current MPA orders conclude with AETC. As I have no expectation that I will get approval for a full public release of the study results in either PowerPoint or Research Paper formats, I wanted to make a series of posts focusing on the major thematic lessons learned instead. All of these are completely unclassified, and won’t include any sensitive use case data or direct descriptions. Ultimately though the real value is in the knowledge gained not the specific assessments conducted.
Understanding the sweet spots for LLM use cases across all domains…
Made famous by the remarks of Donald Rumsfeld in 2002, the quad chart of below can provide valuable insight to the application of LLMs within any domain. There are two continual issues with LLM utilization that both functionally and technically are unavoidable.
This is not a honey pot…this is inception.
Plenty of companies out there pitching custom trained Large Language Models (LLMs), and/or their services to custom train LLMs for the Air Force on our data. To save everyone’s time here’s the absolutely free and publicly accessible crown jewels of the Air Force’s information kingdom. Let the best model win!
An Innovation Contracting Roadmap for Developing AI Systems in Defense Applications
The US Department of Defense (DoD) is at a crucial point in its initial planning stages for how best to deploy capital to develop and purchase AI systems for a range of functions across the services. The plan offered below gives some insight to how the services could leverage the strengths of diverse contracting tools to foster innovation and maintain the DoD's competitive edge. The following plan outlines a suggested order of contract execution and potential parallel implementation of these contract types, to provide both high effectiveness and efficiency of their combined outputs.