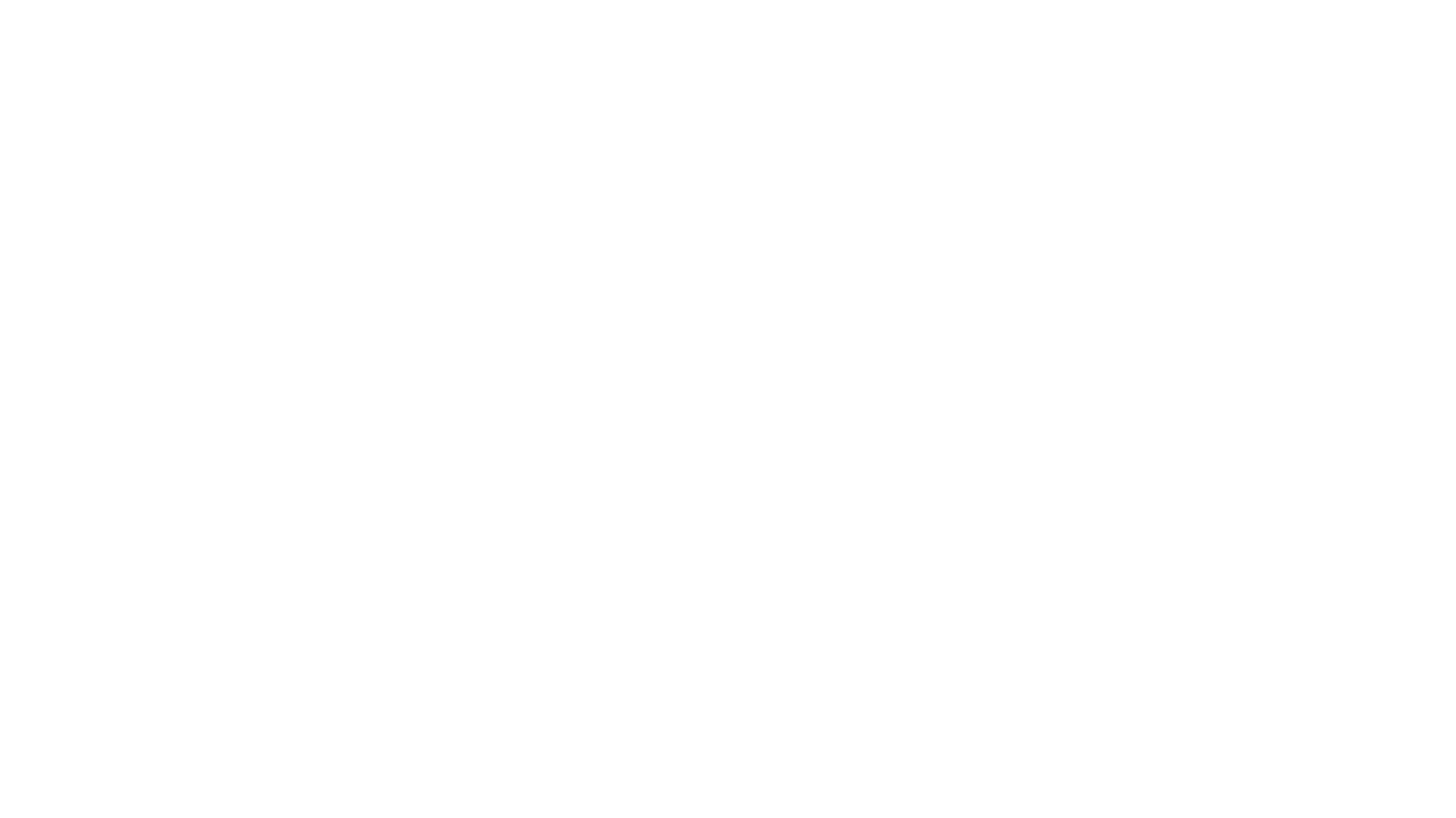
The Education Imperative: Equipping Minds for the U.S.-China AI Race
In a dimly lit Silicon Valley garage, a young coder leans into her laptop screen, her fingers weave lines of code that could one day steer drones through turbulent skies or unlock the tangled code of human DNA. Across the Pacific, in a Shenzhen classroom humming with morning energy, a teenager sketches a neural network on her tablet, her eyes tracing a future where her ideas might reshape cities or cure diseases. These solitary moments, divided by oceans and cultures, share a vital spark: artificial intelligence. As AI redraws the limits of human possibility, the United States and China stand locked in a race not just for technological mastery, but for the minds that will guide its path. Education, once a reliable bridge to stable careers, now faces a pivotal shift, challenged to prepare students for a world where machines think beside us. This journey requires balancing competition with collaboration, all to equip the next generation for an AI-driven age.
AI Supremacy or Bust: Why the U.S. Must Confront China Head-On in the Tech Race
Picture a world where the United States no longer stands at the helm of technological innovation, where artificial intelligence, forged in the crucible of China’s ambition, redraws the map of global power. This vision is not a distant echo from science fiction; it looms on the horizon, growing sharper with every step China takes in the AI race. The stakes reach far beyond the realm of code and circuits, striking at the heart of America’s economic lifeblood and the shield of its national security. In a recent conversation, commentator Saagar Enjeti cut through the noise with a stark truth: “America has decided that our retirement plan is number go up,” a shorthand for how our financial future hinges on the soaring tech stocks that drive our markets (Kantrowitz, 2025). To secure that future, the United States must meet China’s relentless rise with unwavering resolve, a strategy rooted in urgency and a clear grasp of what we stand to lose.
Sanctions, Innovation, and the Global AI Race: Validating “DeepSeek,” Weighing Openness vs. Closedness, and Forecasting Future Dynamics
Introduction and Purpose
The past few years have seen the United States impose export controls designed to prevent China from acquiring advanced AI hardware. These controls restrict or downgrade the capabilities of high-performance processors and specialized chips that are typically used to train and run state-of-the-art artificial intelligence models. Observers initially predicted that these measures would significantly hinder Chinese progress in large-scale AI development (CFR, 2025). The emergence of DeepSeek, an open-source large language model (LLM) reportedly trained on hardware that is less powerful than the latest Western GPUs, has complicated this narrative. DeepSeek’s performance claims include success on coding, math, and language tasks that, according to its developers, match those of proprietary Western models such as GPT-4 (CSIS, 2025). These assertions have not yet been independently verified by recognized benchmarks like MLPerf, which is a global initiative that measures AI system performance in areas such as image recognition, language understanding, and recommendation tasks. Nonetheless, DeepSeek’s story has created renewed discussions about whether sanctions might function as a short-term deterrent but a long-term catalyst for indigenous innovation.
This report examines whether these restrictions have inadvertently motivated Chinese AI researchers to concentrate on more efficient, smaller-scale computing infrastructures. It also explores the tension between open-source and proprietary AI releases, questions the broader geopolitical implications of continued technology controls, and considers how early disruptions in professional job sectors suggest that advanced LLMs may alter traditional expectations of automation. While this document references the original research and uses some of its findings, it focuses on providing a narrative that weaves policy considerations, anecdotes from AI development labs, and forward-looking insights into a cohesive picture of the global AI competition.
Meta's new SAM 2 GenAI Video Model presents MAJOR opportunities for the Defense Industry and militaries all around the world...
The Segment Anything Model 2 (SAM 2) represents a significant leap forward in computer vision and video understanding capabilities, with profound implications for military guidance and surveillance applications. This report provides a comprehensive assessment of SAM 2's potential military applications, focusing on its unique capabilities, likely impact on existing systems, and broader strategic implications.
Updated AGI prediction chart with all the latest info…
Integrated the OpenAI “Stages of AI” levels into the chart to provide a more interesting and detailed projection to the timeline. As noted previously, the “level 2” threshold lines up exactly where OpenAI execs are mentioning in the press that GPT5 will be released. Also of note, if you extrapolate the expected score (GPQA diamond) of Claude 3.5 Opus, it to would fall right near the existing trend-line being released either late this year or early 2025.
What capabilities are coming in GenAI’s near future…
Late last week Open AI internally released their “Stages of Artificial Intelligence”, and it’s worth digging into that a little to highlight the important achievements that are unlocked and stacked at each level.
“How do you do that”, is the common question I often get on my AI projects…
So here’s the simple but EXTREMELY valuable process for drastically improving anything you do with LLMs. First, never zero-shot anything with your LLM usage unless it’s extremely simple tasks you have extreme confidence in the models capability of performing it. Always have the model review/edit its initial output. This is where all the value in the process below comes from. You want to instruct the model how to think, and not just rely on the inate capability of the model to produce the best answer for you. This is especially true the lower down the performance ladder you go with lesser models. In terms of reasoning, there are tons of frameworks and processes, and my current list has 105 across 21 categories. So which are best for what is the magic question to unlocking huge performance gains in output quality from any model.
Revisiting an old problem with a new solution for AI-based Test Question Generation, but this time reverse engineering styles...
Doing AI test question generation from source material is a relatively trivial task these days, but there is a hidden level of complexity to designing the best questions. I had worked this task originally as part of my time in service as part of Air Education & Training Command (AETC) back in 2023. I was able to create viable test questions from source content, but the argument at the time centered around the quality & conformity of the questions relative to existing formats. As the "how" of standardized test question formatting is a closely guarded secret in any training organization, I was working in the blind to all that (to ensure there wasn't any test compromise to existing content) through the duration of my work on the task. That said, I always liked the problem set, and have pondered often about better ways to solve it.
The DoD killer app with GenAI is….training!
What LLMs do best is process existing documentation to produce new text within very complex interpretative instructions. Where that may be an ancillary part of many job workflows in the DoD, the single best fitting and widest impacting task that function represents is training content production. Every service hires very expensive highly experienced SMEs to support the creation, refinement, and publication of training content for every career field in the military. It is by far the most time consuming and labor intensive task for an expensive and limited talent pool. Training commands could leverage LLMs to both speed content creation as well as improve training content quality across all career fields.
AGI by October 2025, that’s the trendline…
Of all the the popular benchmarks used today to test models, I personally think the GPQA Diamond set gives us the most insightful look into predicting the achievement of AGI. The test set is described by its creators in their published research paper as:
Claude 3.5 Sonnet beats Claude 3 Opus in some interesting ways...
With the release today of Anthropic's new top performing LLM, Claude 3.5 Sonnet, I naturally had to run it through some of my recent prompt engineering tests to see how it performed. As this model by many popular benchmarks is supposed to be the best performing model in the world right now, I was curious in what ways this might manifest.
There are two ways to find the highest value integration points for AI in your business…
Not all use cases are high value, so utilizing the right tool for a job is just as much about finding the biggest impact as it is picking the tool for the job. Sometimes using an inverted screwdriver as a hammer to whack a nail into a surface is the right decision. The screwdriver might be the only tool you have on hand with enough mass & volume to attempt the task. Other times you might have plenty of sledgehammers, but it’s just a series of tiny nails going into delicate material that needs the lightest touch possible. The point being that determining the “right” tool for the job requires analyzing the full scope of the task, as it does your situation to determine the best cost/risk/value proposition to choose the best solution available.
The key is backward planning your modular approach to DoD GenAI capability development…
Remember the tech constantly improves regardless of your investment, so start your use case testing on current leading edge closed source models, then acquire and build a modular system that will utilize a future open source model. Use the consistent time lag between the release of leading foundation models to their equivalent open-source competitors to your advantage. With a roughly 6-12 month time gap between the best LLM of today, to the release of an equivalent performing open source model of tomorrow, you can capitalize on both to optimize your DoD project plan.
A fun experiment with GenAI and Mobile devices for the DoD…
Can you use the vision capabilities of multimodal LLMs to detect uniform wear errors? Anyone who’s been through some form of professional military education knows the “joys” of uniform inspection. The only thing worse than having an issue found, is having one found after you, your friend, and someone else already checked your uniform. As there’s a written standard for all uniform wear, the uniforms themselves are all standardized in production, and only personal mobile devices would be required, this all provides an opportunity to test GPT4o and other vision capable LLMs with an interesting challenge.
The big impact of NIPRGPT on the DoD is in risk tolerance not capability…
The NIPRGPT launch provides the first widespread CUI approved GenAI capability for the average military member, but the big landmark is in accepted risk tolerance by the service. I’ll be the first to say it’ll be a challenge to get any significant military use case accomplished by small parameter sized low performance LLM (which is what powers NIPRGPT). As much as this might seem negative, I would argue it is the biggest positive for the technology overall for the DoD. Not all GenAI systems or applications are high threat, and as such a graduated scale should be applied to their evaluation and approval. Starting small and proving that fact is VERY important, because what establishing a graduated risk scale relative to model/system capability does is ultimately raise the floor of mass capability deployment.
Solving the word count problem with LLMs, a continuation of the sub-token solution…
So unlike the previous solution in my other post about “solving for R”, this solution seems to have a minimum model threshold performance with small models. This solution was tested with one shot initial success on all of the following models: Mistral Large, Llama3-8b-8192, GPT3.5, GPT4o, and Claude 3 Sonnet. Interestingly, Claude 3 Haiku and Opus models were the only models to not separate the “small…and” into two separate words, therefore leaving their counts off by one word. Also of note, if your run the sample paragraph through online word counters, they too will count only 54 instead of 55 words.
Solving for “R” with lower quality models, the challenge and the solution…
A popular observed issue with LLMs is their perceived inability to solve simple counting problems, but their are ways to solve these issue if you understand the inherent system functions that are causing the errors. The current problem/test is counting the number of times a letter occurs within a given word. The core issue is that in the vectorization of the tokens, letters that are the same and next to each other are getting lost in translation of the mathematical conversion. The solution is to make the individual target variables (letters of the target word) long enough to take up full token lengths, so their proper representation is not lost in vectorization and computation by the model.
This kind of ignorance just annoys the crap out of me. Here’s the simple prompt engineering fix to these type of problems with GPT4o. It’s literally one extra prompt. 🙄
Prompt Solution for Model counting issues (solutions for small and large models)
If I was Google and I wanted to make a big dent in the defense space with a ground breaking research project, this is what I would do...
The core thesis would be MuZero applied to "Command: Modern Air/Naval Operations" to develop unique winning game strategies/tactics, with the winning gameplay videos being analyzed by Gemini Pro 1.5 to explain in detail the applicable strategies/tactics for reporting. Google's Deepmind has already shown their MuZero model is capable of mastering basic video games, so upscaling that to one of the most complex military battle simulators would be a interesting challenge. The value being that MuZero and all previous Alpha models have both mastered their target games, and in doing so developed new and valuable strategies and tactics to accomplish those feats. Where humans were utilized previously to analyze the applicable gameplay results to interpret the critical winning moves of the overall gameplay, automating this complex task with Google's new multimodal modal would be another equally interesting challenge.
GPT4o does a hell of a job with audio narration, and really DESTROYS the whole business of ElvenLabs and other similar companies...
I had a feeling after watching the launch demos of GPT4o that you could use this new model for audio narration purposes, and forgo entire separate paid audio narration services like ElevenLabs. I have been very impressed with what ElevenLabs has provided up to now, with the money you pay being well worth their output, but why keep paying for a separate service with you have GPT4o? The video below is just the first test of my audio narration experiment using the new ChatGPT iOS app with voice features. It's a 5 minute narration of the intro and part of the first chapter story from my upcoming new book. This fictional story plays out in sections at the end of each chapter of the book as a fun hypothetical employment of the chapter content.