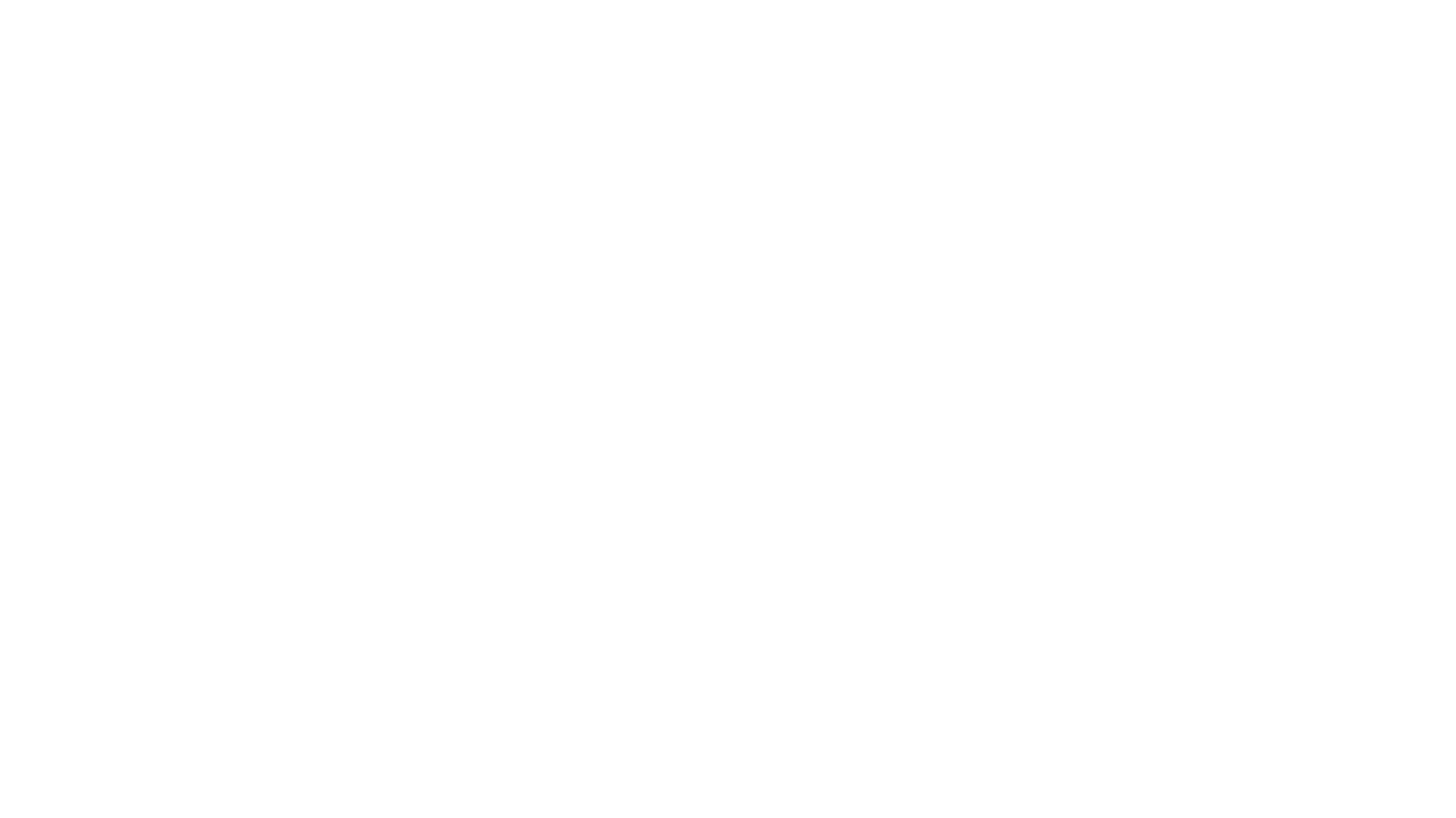
Has anyone thought to try using GPT4o to observe, direct, or hypothesize on lab experiments?…
With the new multimodal and rapid interactions capable via a smartphone, it begs the question of what could you use it for beyond simple conversations. Without jumping straight to building skynet, it would be an interesting experiment to let GPT4o interact with real world lab experiments via observation and communication via a smartphone. Setup a tripod live stream the video of your lab table experiment, interact with the model live during the experiment via chat, and just see how it goes.
I really look forward to doing more cool experiments with GPT4o…
There’s not a ton of additional overall quality you get from GPT4o vs GPT4, but there is a lot more possible use case applications and speed as well that you do get. GPT4o is very obviously tuned for brevity to better enable conversional interactions, but the multimodality does seem to provide improved results on some older more problematic use cases. Image generation of characters across multiple poses while maintaining the same character look/style has improved. This would be really interesting to play with more for all kinds of story illustrations purposes. I really want to revisit my original handwriting analysis use cases from last year with GPT4V, as there were some really impressive demos of handwriting creation via image generation with GOT4o from OpenAI.
When you get a new toy, why not go all out and test the limits, and that exactly what I did with GPT4o...
I still need to finish the longer educational presentation of how my complex GenAI workflow functions, and all the dissection of the various components, but I have released the latest and most complex version for problem solving. So what is now available at my website linked below, is an attempt at optimizing generalized workflow concepts with advanced prompt engineering techniques and structures utilizing task customizable analytical framework implementations. That's just a lot of word salad to say this is a complicated way of using a customized and complex approach to problem solving with GenAI. You can throw just about any kind of problem you want through the workflow, but for the purposes of testing I find using various news articles describing complex real world issues a fun use case.
I have spent way too many hours messing with getting this working, and now it's time for sleep...
Getting LLMs to draw complex objects, in this case a detailed turtle, using nothing but html code without image files is REALLY hard. This is about as good as I could get tonight, and as simple and silly as that looks, it's miles ahead of the zero short results of any model without using the workflow concepts I have been running on about recently.
Here's for the folks who asked for the version of my workflow for fiction editing...
To ensure that I answered the mail to the handful of people that contacted me asking about the fiction focused version of the workflow I shared yesterday, it is shown below. I'm going to devote some time this weekend to cleaning both up into full guides with step-by-step templates, so look for those updates on my website soon.
It’s quite the impressive result when you finally solve a complex prompt engineering workflow, but it’s also generated a ton more work for me…
Just when I thought I had finally neared completion of editing my book, I also finally achieved full success on my GenAI manuscript editing workflow, and damn did it work. It’s both an amusing moment to solve a series of problems that unlock a huge capability, but also sort of frustrating to realize how much writing/rewriting I still have left in my book. The results of both of those situations are ultimately very positive for the book and all my future GenAI workflows. For context, my previous editorial workflows were generating maybe 1-2 pages of notes, that were mainly just resulting in positive reflections of the current content with a few suggestions for content improvement. The new workflow breakthrough yielded over 8 pages of extremely detailed and high-quality feedback that was fantastic! It really was one of those magical “AH-HA” moments of both achievement and realization.
Are you using the right analytical framework for your tasks in your GenAI prompts?…
There are usually multiple ways to accomplish the same task, as well as there’s a large amount of preexisting information on the many and optimized ways to analyze data. Are you using the best productivity and/or analytical frameworks for your tasks? Do you know what those options are? Are you choosing and alternating frameworks for each task to optimize results for the specific task?
Lessons to learn from the sUAS market for the growing GenAI market in the defense sector…
Thinking back seven years ago to when I was helping run the Thunderdrone event at SOFWERX, there’s a number of interesting lessons to be learned for how the sUAS market evolved from that point, and how those lessons might apply now to the newly growing GenAI market. “We can’t even talk about testing these things with weapons on them”, was the cry from so many DoD leaders at the time (ISR was the primary focus). Security and safety was the top priority regardless of the weapons potential. “We need better, bigger, and more capable platforms”, was the constant drum beat of requirements managers at the time (Group 3+ was the focus for more payload and flight time). Looking forward to today’s battlefield, we see quite the opposite.
The core limitation with current GenAI systems is they mandate human curation of complex formatted data production…
It’s a lived technical reality for a few, but not necessarily an obvious realization for many GenAI observers, that the current state of the technology limits the model’s interaction with data to complete interactions only. In simple terms, the models cannot currently interact outside of themselves with separate applications to affect pieces or parts of large data files or applications. A basic example would be the models could perform a grammar check on a document, but not automatically interact with MS Word to fix only the errors it identifies. You would either need to have the model attempt to rewrite the entire (or some whole section of) text to fix the errors (hoping to note induce more in the process), or you would have to manually fix the errors separately yourself. We saw this same thing with diffusion models that generate images until recently, when notable major diffusion model providers started adding additional tools to their user interfaces to allow image subsection alterations. Soon with agents we will see similar capabilities come to LLMs.
Think I might write another book, but this time with more GenAI assistance…
I have been excited with all the ways that GenAI tools have been able to help me edit my current book, and it excites me about what more I could do. I really want to go back revisit the concept I wrote about in a post last year regarding using video interviews as a source of analysis for insights. Specifically I was thinking about writing a book or maybe a short series of articles on the evolution of battlefield technology in the Ukraine war. There is ample content in the form of online interviews on this topic, from the front lines to the development labs. Not that there is a shortage of existing written content that discusses this topic, but that’s mostly the point. To see how fast it is possible to pull together meaningful long form content from a larger source repository of audio-visual content.
PROTIP for DoD GenAI system approval: Isolate your prompts to buttons instead of using free text fields…
There was much gnashing of teeth recently at an early look into the upcoming DAF GenAI system approval process, but instead of complaining, there’s some tricks to system design you can use to help improve your odds of approval. First, one of the biggest issues with any GenAI system is the ethics considerations. Drawing the line on what is and isn’t allowed in or out of the model for use is a very tricky thing. Ultimately some line must be drawn by someone, but therein lies the issue. How does the system owner enforce that limitation?
With the release yesterday of the new Llama 3 LLMs from Meta, we are right on track with my predictions from last year’s GenAI study for the DAF…
As of today, we now have open-source LLMs that are at least a 10th as small, but approximately as capable as the original release of GPT4 last year. So, in roughly a year we have seen development in GenAI progress to the point where the baseline standard for open-source LLMs (Meta’s Llama models) catches up to the original groundbreaking foundational model standard of previous year. Now the interesting thing is that this achievement isn’t actually new. We saw the exact same thing last year with the release of Llama 2, as it was roughly equivalent to GPT 3.5 but smaller and released roughly a year after as well. So, this is just more validation of the existing developmental trendline within the field. But the interesting thing isn’t where we are today on that trendline, but where we are all going very soon.
One of the most important long-term outcomes of the rapid growth of Generative AI, isn’t AGI or ASI, it’s regulatory changes to allow small modular nuclear power…
Regardless of your belief that the scaling of Generative AI development will ever achieve a particular extreme computational performance level, one thing that everyone should agree on is that many companies will keep voraciously pursuing more compute resources to at least keep trying. That rapidly scaling compute is extremely power intensive, and data centers can’t just go anywhere due to the limitations of power transmission with current material science. Those data centers also require very stable baseload power at very large capacity, often approaching multiple megawatts or more. Even if you could get around all the environmental and political issues with fossil fuel-based power generation, it’s still bound by the geographic constraints of the natural resource sources that make placing the power plants tricky. The only option is nuclear power for high output capacity, stable, widely deployable power generation.
With no big deployments of GenAI capabilities coming soon in the DoD, one of the best efforts that could be done in the meantime is prompt engineering…
The DoD has plenty of internship programs, active service volunteer efforts, and myriad of other methods to bring together a team of personnel to pursue prompt engineering on any number of existing platforms. In fact, this has already been done by DAF's CAITO last year in a small-scale effort to produce the first version of a prompt engineering handbook. The core issue is it could and should be an enduring effort focused on personnel training through direct experience. What better way to train the force than through direct experience of rotating participants. Any platform or model can be used, any data or use case could be utilized, and anyone could be a participant. Prompts are the ubiquitous and common tool all models utilize for function, and they can be continuously developed, tested, and cataloged for the evolution of capability across the force.
Capturing the efficiency gains of LLM’s starts with and is centered on your ability to build and implement prompt based workflows…
Simply put, you need to build up your experience around using individual prompts as building blocks to construct the larger effects which you then orchestrate into complete workflows to produce high quality outputs. You do not need private models, specially fine tuned models, or super secure 3rd party services to do any of this type of development for your organization. You could literally have an unpaid college intern do this for you over a few weeks or months with nothing more than a $20/month ChatGPT or Claude account. But what does this development look like?
Have you jumped on the Generative AI train with your business yet, or are you just letting your competitors leave you behind?
If you aren’t yet utilizing GenAI to the maximum extent possible to grow your business's output, I can guarantee you your competitors currently are. The one place above all there is a ton of impact, both in amount and quality of output with GenAI, is in marketing and business development. Rather you need to create marketing materials, write a proposal, or just increase the efficiency of basic analytical support tasks, there is a GenAI tool out there to help you accomplish it. You can argue the effectiveness or the quality of the current state of these tools, but two simple facts remain. First, that the tools do and will continue to get better over a surprisingly short amount of time. Second, rather your company chooses to utilize them or not, some other company (or many) will, and that will give them an ever-growing edge in operational efficiency and business growth. The question for you then becomes, when and what GenAI tools should you use to grow your own company’s edge in the market?
The senior leaders of the DoD have been fond of pointedly asking, “How are LLMs going to help us do warfighting?”…
The simple answer is LLMs are a foundational technology to allowing and enabling the advanced training and operation of robotics. Rather you want to point to any number of the forms of human workload offset or replacement, robotics will make a major impact in the years to come. This video of a recent presentation done by Nvidia researchers should hopefully open people’s eyes to what’s coming, and what’s possible even now.
The release of Grok-1 marks the next watershed moment for GenAI for the DoD, with better than GPT 3.5 level of performance, but most importantly with an unrestricted license and model…
For the defense sector, GenAI has been a bit of a waiting game on multiple fronts, but one of the biggest issues just got addressed. What Grok-1 represents is a golden opportunity for defense products and projects to utilize an open-source model for virtually any use case that it wants/needs. The model was released under the Apache 2.0 license, which is one of the current gold standards for open-source use within the defense sector today. Also, the model itself was not fine-tuned in any way, which again for defense purposes is very advantageous, as many use cases would or might require outputs from the model that would bump up against guardrails imposed by the originating commercial or civilian developer. Combine all that with an overall performance quality level exceeding GPT3.5, and you have something truly special.
Class leading performance in math, even larger context windows, improved accuracy across all metrics, and multiple model sizes solidifies Anthropic's role as a leader in GenAI...
The best just keep getting better, and though I do really like the multi-modality of ChatGPT with Dalle3, Claude just keeps setting the standard for pure textual content generation with their new version 3 models. The link below highlights Anthropic's published metrics on the performance of their new model versions, and as expected they are damn impressive. Of note, Claude is the competitive tool of choice for AWS in the ongoing market battle with Microsoft and their offerings from OpenAI. If I had to choose just one, at this point I might go with Claude, just for the amount of textual based usage I do. Also might be an interesting safety play given the uncertain implications and outcomes of the current lawsuit that Elon Musk filed against OpenAI.
Why is there no significant investment in GenAI in the DoD to date? This panel was a masterclass in the answer…
In short, Machine Learning is currently viewed as the more worthy investment so it gets the focus, and GenAI is a wonderful thing for our adversaries to waste their money on pursuing. Agree or disagree, that was the doomer message coming from experts on the panel, leaving only the commercial reps to try and salvage some kind of worth to pursuing the technology in a meaningful way within the DoD.